Generative AI is a new type of artificial intelligence that has the potential to revolutionize the way we work.
It can be used to automate tasks, generate new ideas, and improve efficiency. In this blog, we will explore the ways in which generative AI could create value for businesses and the potential impact on workers in the fashion industry.
"Through the lens of generative AI, brands see not just what is, but what can be."
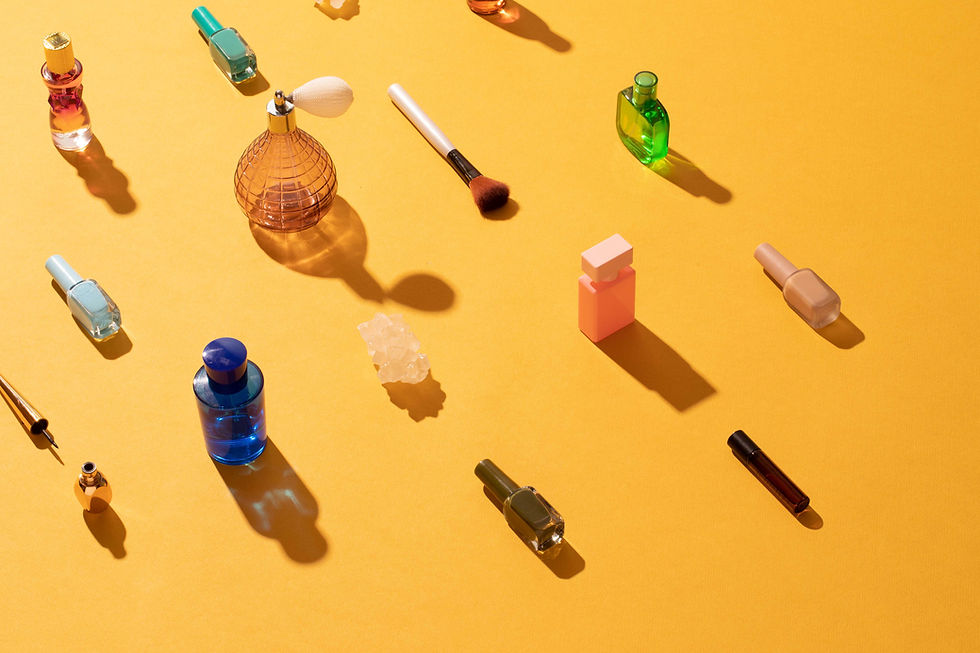
How is Generative AI Being Used in the fashion Industry Today?
Generative AI has the potential to add between $2.6 trillion and $4.4 trillion to the global economy each year across the 63 use cases we analyzed, according to McKinsey & Company. This is more than the entire GDP of the United Kingdom in 2021.
The fashion industry stands as a key beneficiary, employing generative AI for product design, personalized marketing, and supply chain optimization, as noted by CB Insights.
Sustainability is a major area where generative AI can make a lasting impact. It enables the design of eco-friendly products and enhances production efficiency to minimize waste.
Examples of current implementations:
H&M uses generative AI for trend-responsive clothing designs.
Nike employs it for data-driven, cost-effective production planning.
Zalando leverages AI for customized product recommendations.
ASOS utilizes generative AI for virtual try-ons, reducing returns.
Stella McCartney applies AI for designing sustainable materials.

Generative AI offers compelling applications in fashion, ranging from design innovation and operational efficiency to sustainability and personalized customer experiences.
Generative AI is already being used by brands in the fashion industry today in a variety of ways, including:
Design: Generative AI can be used to generate new designs for clothes, shoes, and accessories. This can help brands to be more innovative and to keep up with the latest trends.
Manufacturing: Generative AI can be used to optimize manufacturing processes and reduce costs. For example, generative AI can be used to design more efficient production lines and to identify areas where waste can be reduced.
Marketing: Generative AI can be used to create personalized marketing campaigns and reach customers more effectively.
Such as, generative AI can be used to generate personalized product recommendations or to create targeted social media ads.
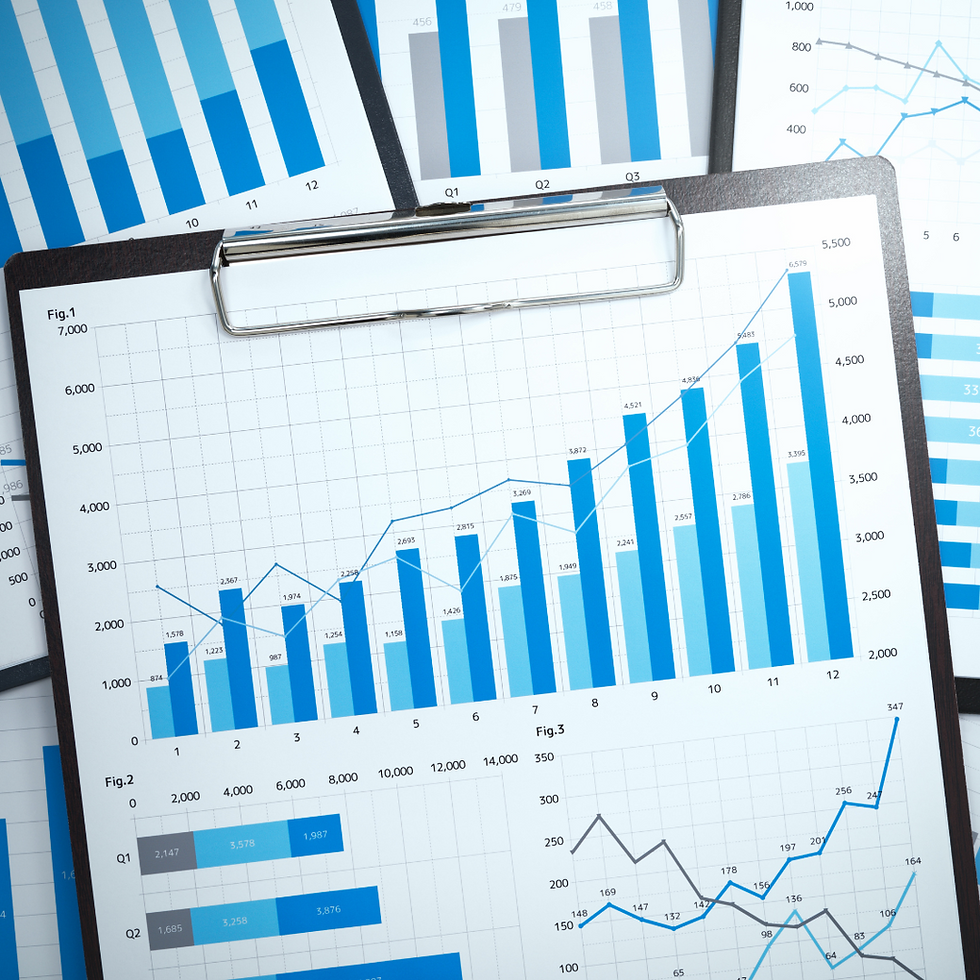
Sales: Generative AI can be used to improve the sales process and increase sales. For example, generative AI can be used to power virtual try-on features or to develop new customer loyalty programs.
Sustainability: Generative AI can be used to design more sustainable products and materials. Generative AI can be used to design products that are made from recycled materials or that are less water-intensive to produce.
In addition to these examples, generative AI is also being used by fashion brands to:
Create virtual try-on experiences
Generate personalized marketing campaigns
Improve customer service
Reduce waste and environmental impact

The Potential Impact of Generative AI
The potential impact of generative AI on the fashion industry is transformative, offering a blend of technological innovation with creative design.
Design Innovation
Generative AI can automate the design process, rapidly generating numerous creative patterns and styles. This accelerates the time-to-market for new collections and allows designers to focus on refining and curating AI-generated concepts.
Personalization
With generative AI, fashion brands can offer unprecedented levels of customization.
Consumers could input their preferences and receive tailored product recommendations, or even custom-designed clothing, enhancing customer engagement and satisfaction.
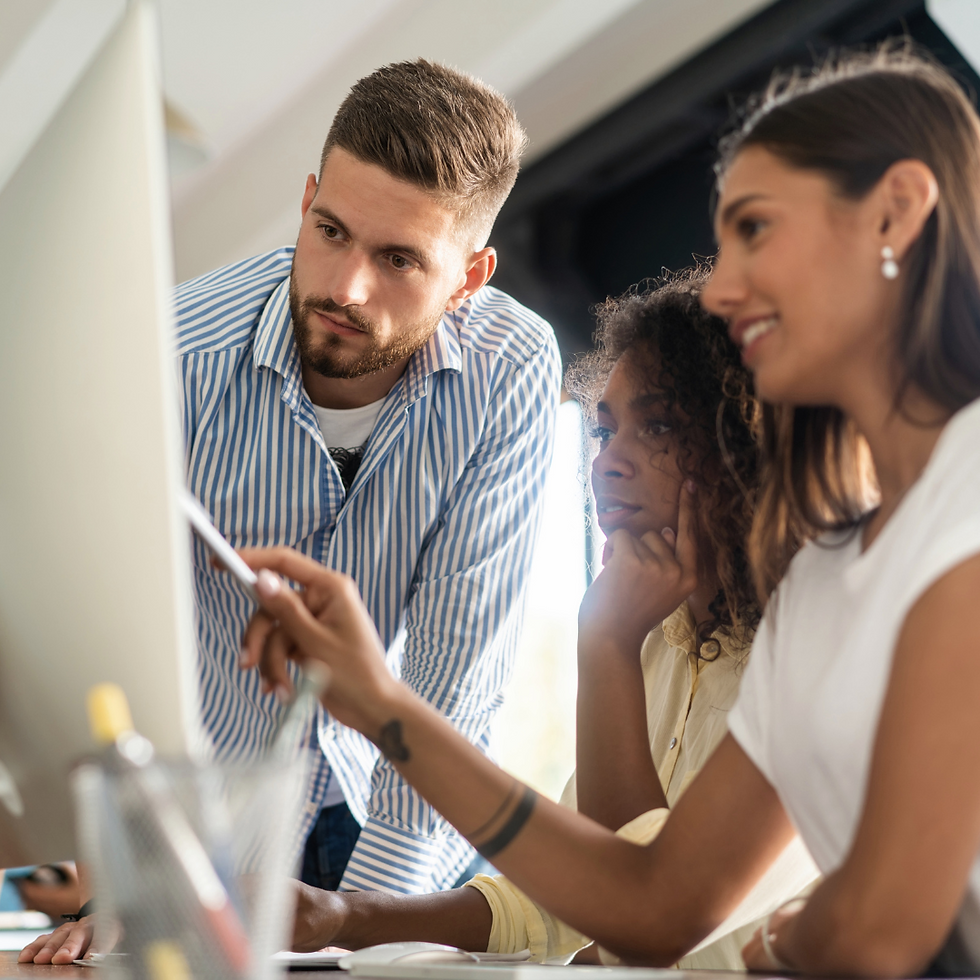
Sustainability
Generative AI can contribute to the industry's growing focus on sustainability. By optimizing designs for material usage and recommending eco-friendly materials, AI can reduce waste and the overall environmental footprint.
Supply Chain Optimization
From inventory management to production planning, generative AI can provide data-driven insights to streamline operations. This not only reduces costs but also enhances responsiveness to market trends and demands.
Marketing and Sales
AI-generated personalized campaigns can significantly improve consumer engagement. Algorithms can analyze customer behavior to tailor marketing strategies, thus driving sales and enhancing customer retention.
Competitive Edge
Early adoption of generative AI can offer brands a significant competitive advantage, allowing them to innovate faster, reduce operational costs, and meet customer needs more effectively.
Generative AI has the potential to revolutionize the fashion industry, affecting everything from design and production to marketing and sales, while also contributing to sustainability efforts.
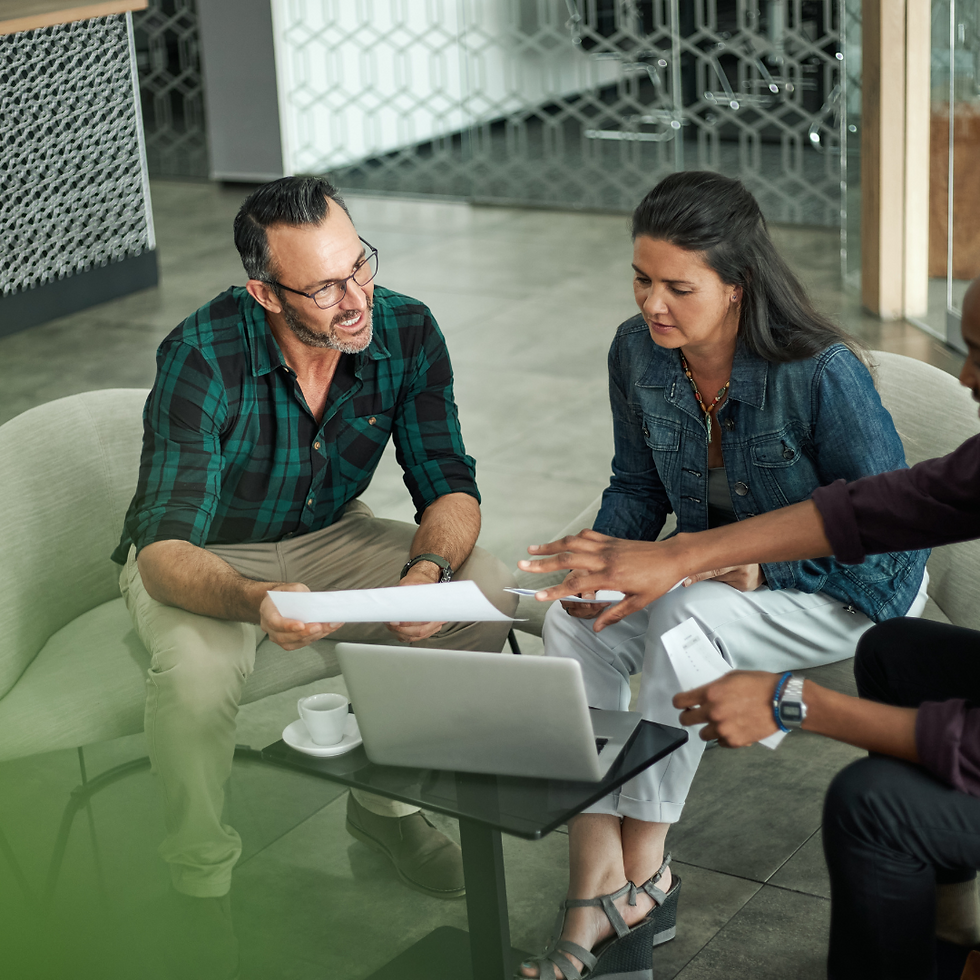
Case Studies of Brands Using GenAI
H&M: Designing Trend-Responsive Collections
H&M deploys generative AI to create new designs, reducing the time it takes to get products from concept to customer.
The company feeds a dataset of its past collections and current fashion trends into a generative model, which then crafts new designs.
The resulting designs align well with anticipated consumer preferences, enhancing the brand's agility in a fast-paced market.
Nike: Streamlining Production Efficiency
Nike uses generative AI to bring down production costs and elevate efficiency.
The AI model is trained on historical production data and real-time market trends.
It subsequently generates production plans optimized for cost-effectiveness and speed, allowing Nike to dynamically adjust its production strategies.

Zalando: Personalized Shopping Experiences
Zalando focuses on consumer personalization through generative AI.
Their model analyzes customers' purchase history and online behavior to deliver individualized product recommendations. This hyper-personalization serves to increase consumer engagement and conversion rates.
ASOS: Virtual Try-On for Informed Purchasing
ASOS employs generative AI to enhance its virtual try-on feature.
The AI simulates how different items would appear on customers, helping them visualize their choices without physically trying them on.
This capability not only improves customer satisfaction but also minimizes the likelihood of returns, thereby reducing operational costs.
Stella McCartney: Crafting Sustainable Materials
Stella McCartney utilizes generative AI to pioneer new, environmentally-friendly materials.
Their AI model sifts through data on current materials and their environmental impact, generating designs for new materials that fulfill both aesthetic and sustainability criteria.
GenAI is finding diverse and impactful applications across the fashion industry, serving to accelerate design, refine production, enhance customer engagement, and promote sustainability.

Benefits of Using Generative AI
The use of generative AI in the fashion industry is not just an interesting intersection of technology and creativity; it also offers a wide array of tangible benefits.
Enhanced Creativity
Automated Brainstorming: GenAI can produce a plethora of design ideas in a short amount of time, providing designers with creative inspiration.
Combining Styles: The technology can fuse different fashion eras or types, creating unique styles that a human might not easily think of.
Cost-Efficiency
Material Utilization: AI can optimize patterns to minimize fabric waste.
Speed of Prototyping: It is easier and faster to make changes to a virtual design, saving both time and resources.
Customization
Personalized Designs: AI can create one-of-a-kind pieces based on individual customer preferences, which can be a significant selling point.
Fit Optimization: Generative AI can help create garments that are optimized for different body types and sizes, improving fit and customer satisfaction.
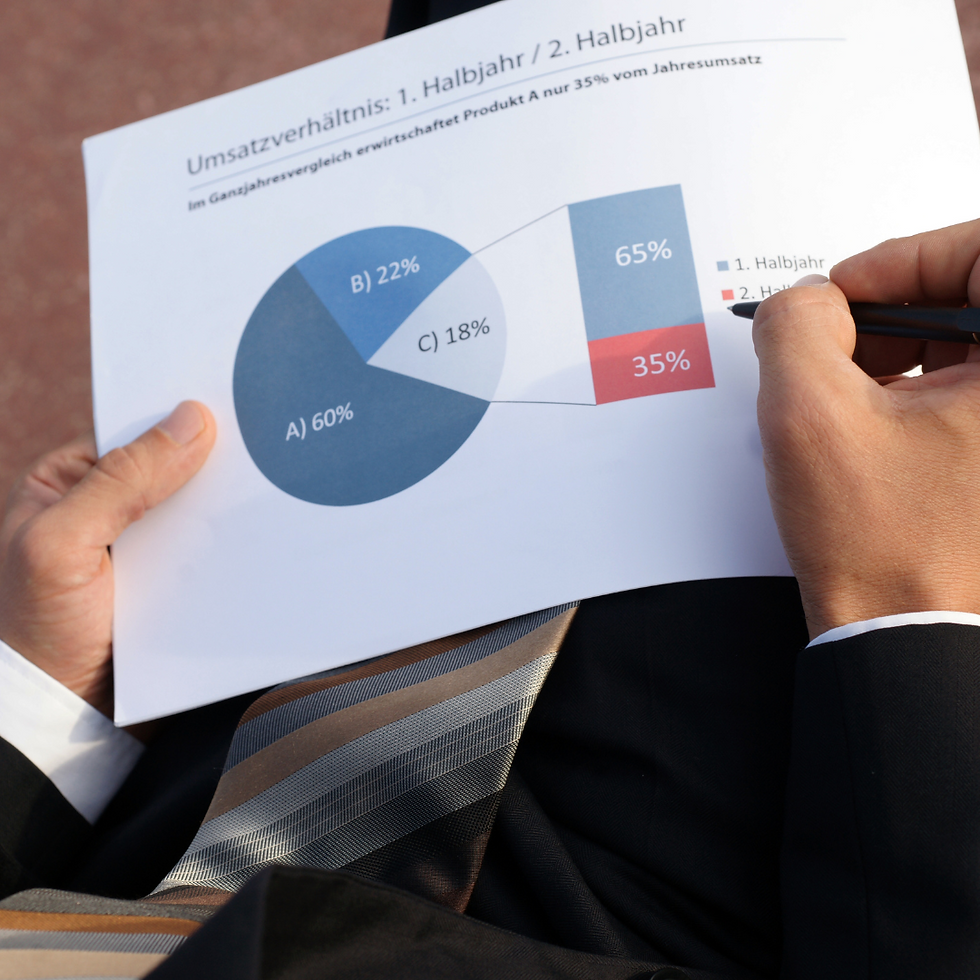
Sustainability
Reduced Waste: By optimizing design and fit, AI can contribute to reducing the overall waste in fashion, including material scraps and unsold inventory.
Data-Driven Sustainability: AI algorithms can help in selecting sustainable materials and manufacturing processes.
Market Research and Consumer Insights
Trend Prediction: AI can analyze large sets of data to predict future fashion trends, helping designers and retailers stock up on inventory that is more likely to sell.
Consumer Behavior Analysis: AI can analyze customer interactions with virtual designs to gauge market reception before physical samples are produced.
Automation and Efficiency
Streamlined Manufacturing: AI can translate designs directly into machine-readable formats, speeding up the manufacturing process.
Quality Control: AI algorithms can automatically identify defects or inconsistencies in materials or final products, ensuring a higher level of quality.
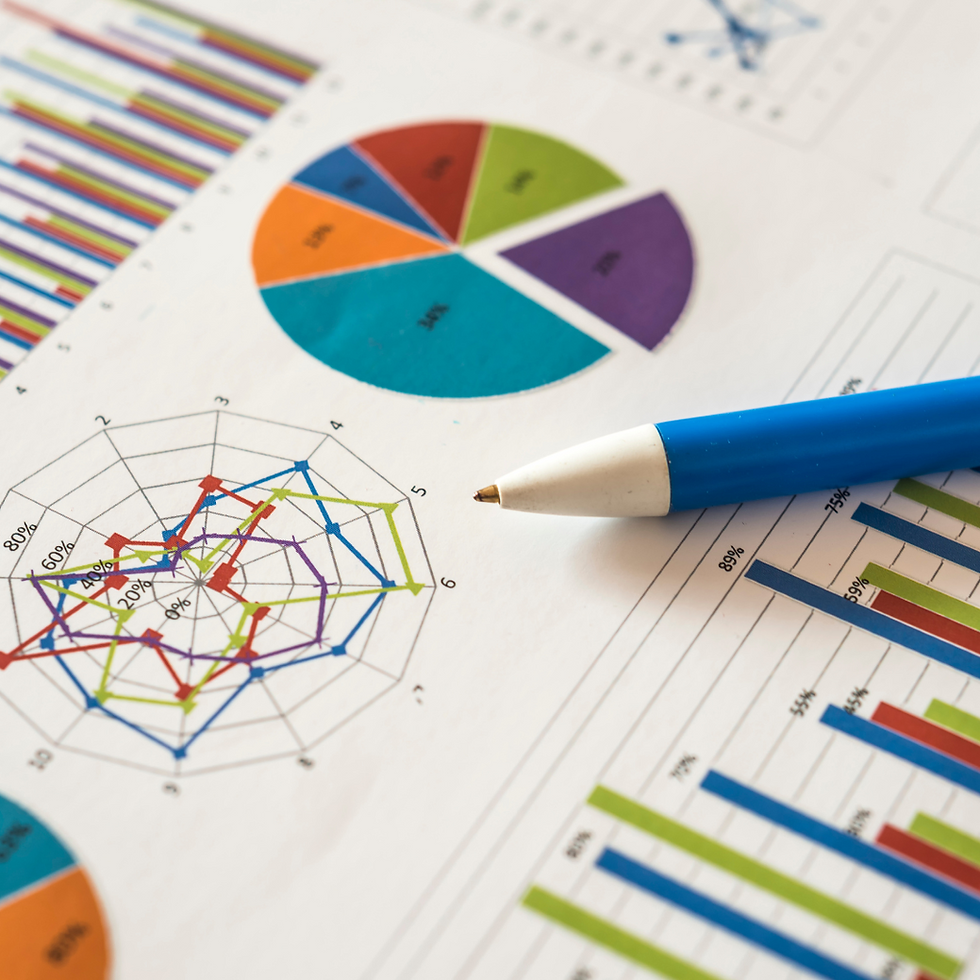
Global Reach
Localized Designs: AI can analyze regional trends and preferences to create designs that are tailored to specific markets.
Instant Translation: GenAI can help quickly adapt a successful design into multiple variations to suit different cultural norms or weather conditions.
Real-time Adaptability
Seasonal Shifts: AI can adapt designs in real-time to account for sudden shifts in weather or trends. Inventory Management: By analyzing sales and inventory data, generative AI can assist in the creation of designs that fill identified gaps in the product lineup.
Accessibility
Democratizing Design: With AI tools becoming more accessible and user-friendly, smaller brands and independent designers can also take advantage of advanced design capabilities.
The implementation of generative AI in the fashion industry is an exciting development that can result in a more innovative, efficient, and customized approach to fashion design and retail.
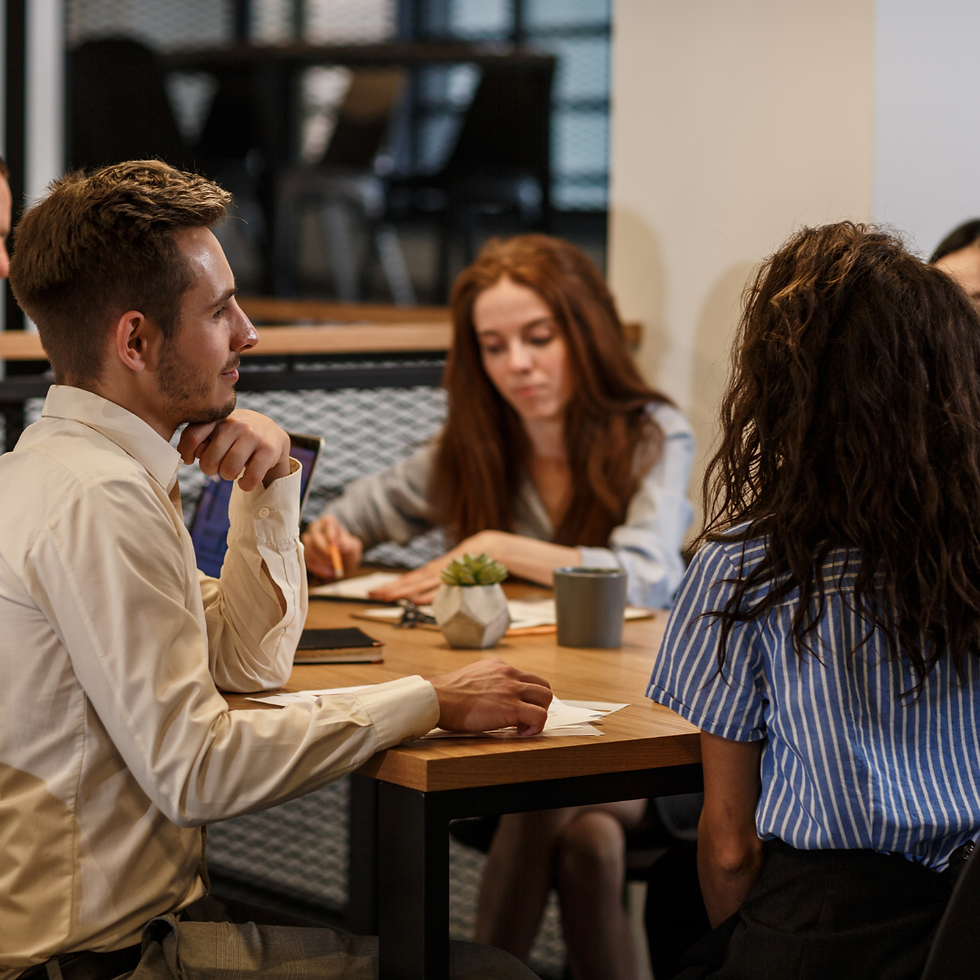
Challenges of Using Generative AI in the Fashion Industry
GenAI has significant potential to revolutionize the fashion industry, there are also numerous challenges that need to be addressed for successful and ethical integration.
Ethical Concerns
Intellectual Property: Generative AI might inadvertently produce designs that closely resemble existing ones, thereby raising copyright issues.
Loss of Jobs: Automation via AI could result in layoffs, particularly for low-skilled jobs in areas like pattern-making and quality control.
Technical Limitations
Computational Costs: Generative AI models are resource-intensive, requiring significant computational power, which could be expensive.
Data Quality: Accurate and meaningful output depends on high-quality, unbiased data, which is not always easy to obtain.
Creativity and Originality
Human Touch: There's a concern that AI-generated designs might lack the emotional and cultural nuances that a human designer would add.
Over-Reliance: Heavy dependence on AI for creative processes could stifle human creativity and intuition.
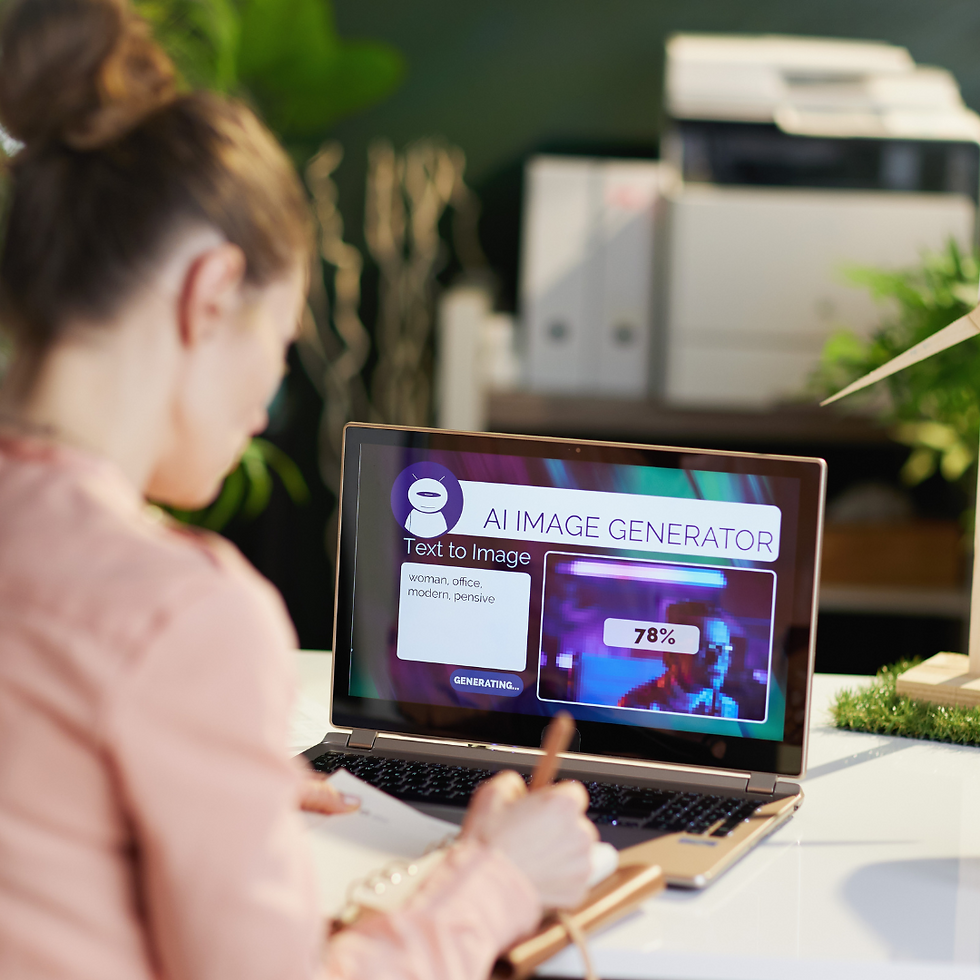
Complexity and Usability
Skill Gap: Operating advanced AI models requires a level of technical expertise that many in the fashion industry may not have.
Interpreting Results: AI might generate designs that are technically feasible but not practical or aesthetically pleasing from a human perspective.
Economic Implications
Initial Costs: Setting up the necessary infrastructure for AI can be prohibitively expensive for smaller brands or independent designers.
Monopoly Risks: The high costs and technical expertise required may lead to a monopolization by big players who can afford these technologies.
Quality and Consistency
Standardization: Translating AI-generated designs into physical products may face issues due to lack of standardization in manufacturing processes.
Quality Control: Machines might not catch subtleties and details that a human inspector would, leading to quality issues.
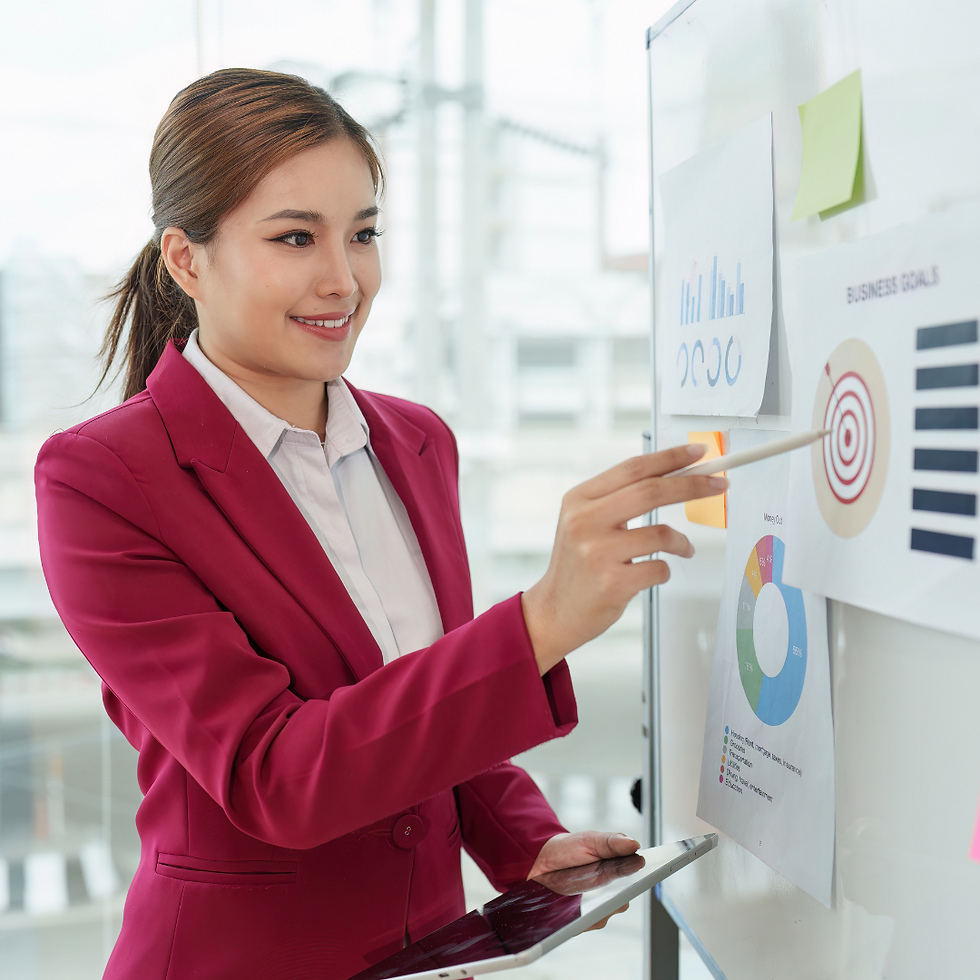
Sustainability
Energy Consumption: AI models require significant energy, which could be a concern for an industry increasingly focused on sustainability.
Material Waste: Generative algorithms might not always optimize for material usage, possibly leading to waste.
Cultural and Social Factors
Cultural Sensitivity: Algorithms might inadvertently generate designs that are culturally insensitive or inappropriate.
Consumer Skepticism: There might be resistance from consumers who prefer human-designed products for their emotional or artistic value.
Regulatory Challenges
Data Privacy: The use of consumer data for personalized designs could raise privacy concerns.
Regulatory Compliance: As AI is an emerging field, the regulatory landscape is still uncertain, and non-compliance could lead to legal issues.
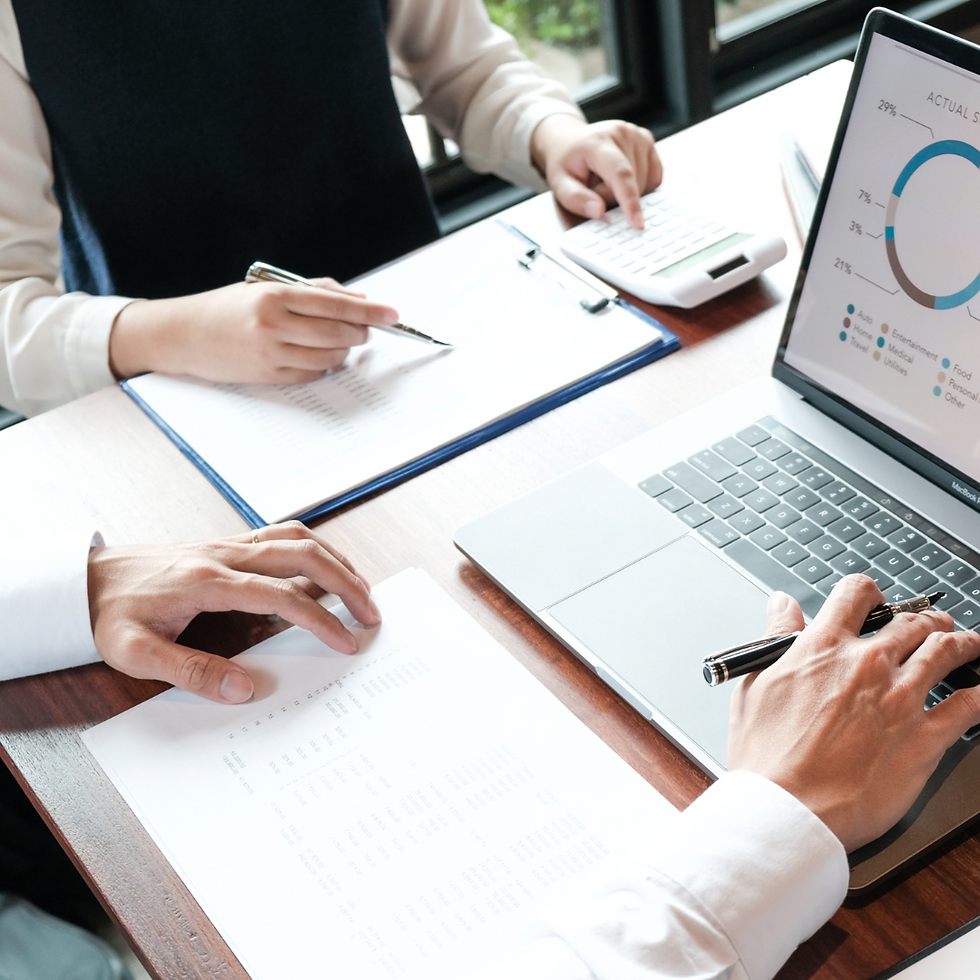
Market Adaptation
Fast-Changing Trends: The rapidly changing nature of fashion might outpace the ability of AI systems to adapt quickly.
Consumer Trust: There may be initial hesitation in accepting AI-generated designs, affecting market adoption rates.
While generative AI has the potential to offer remarkable innovations in the fashion industry, overcoming these challenges will require a multidisciplinary approach, involving stakeholders from technology, fashion, law, and ethics.
Ethical Considerations for Using GenAI in the Fashion Industry
The integration of generative AI into the fashion industry brings about a series of ethical considerations that stakeholders must grapple with.
Ensuring that AI serves to enhance rather than diminish the industry's ethical standing is crucial. Here are some of the key ethical considerations:
Intellectual Property and Originality
Design Similarity: Generative AI may unintentionally create designs that are similar to existing human-created designs, posing questions about plagiarism and originality.
Ownership: Determining who owns the copyright for AI-generated designs—be it the AI developer, the user, or a shared ownership model—is a complex ethical and legal issue.
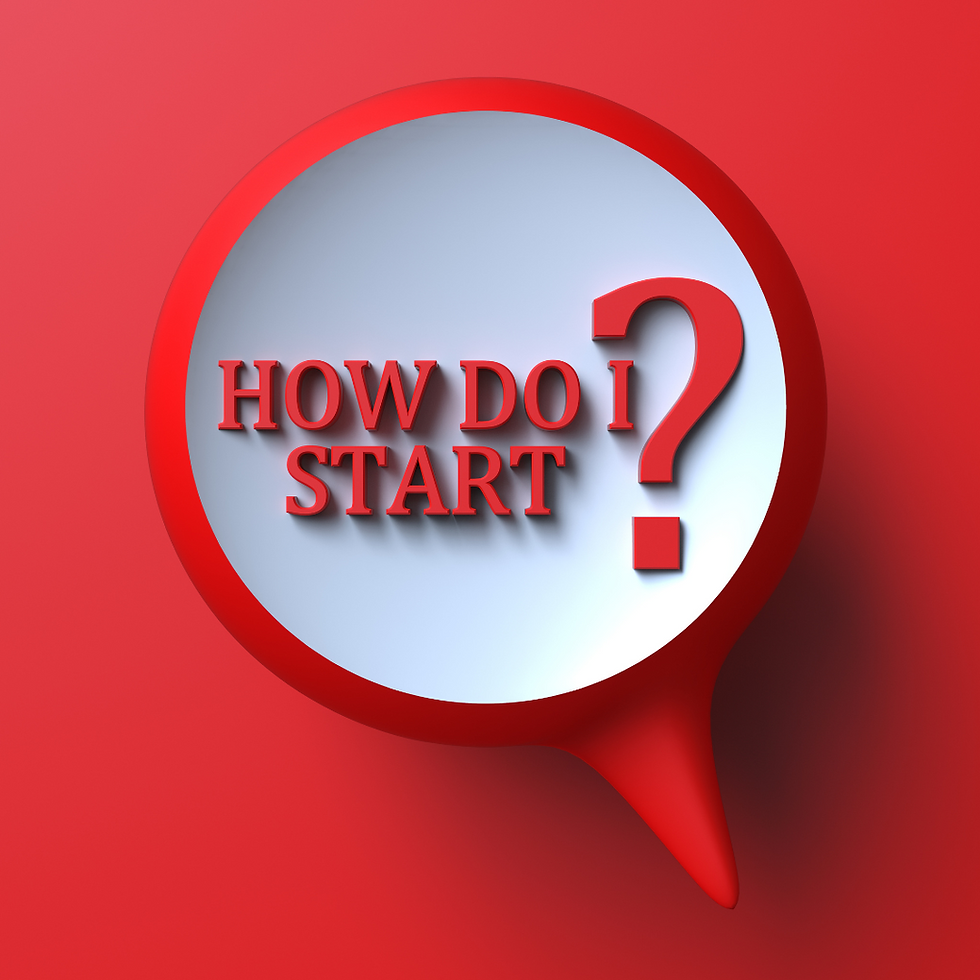
Labor and Employment
Job Displacement: The automation of various aspects, from design generation to quality control, may result in job losses. The ethical implications of this must be managed carefully.
Upskilling: Ethical deployment may require retraining programs for employees who lose their jobs to automation, to help them adapt to new roles.
Data Ethics
Data Collection: The use of consumer data to tailor designs poses questions around privacy and data ownership.
Bias: If the training data includes biased human decisions—such as a focus on a narrow set of body types—AI may perpetuate or amplify these biases.
Sustainability
Energy Usage: AI's high computational requirements could contribute to environmental degradation, countering the fashion industry's efforts towards sustainability.
Waste: The ease with which AI can generate designs might lead to a culture of overproduction, contributing to material waste and further environmental harm.
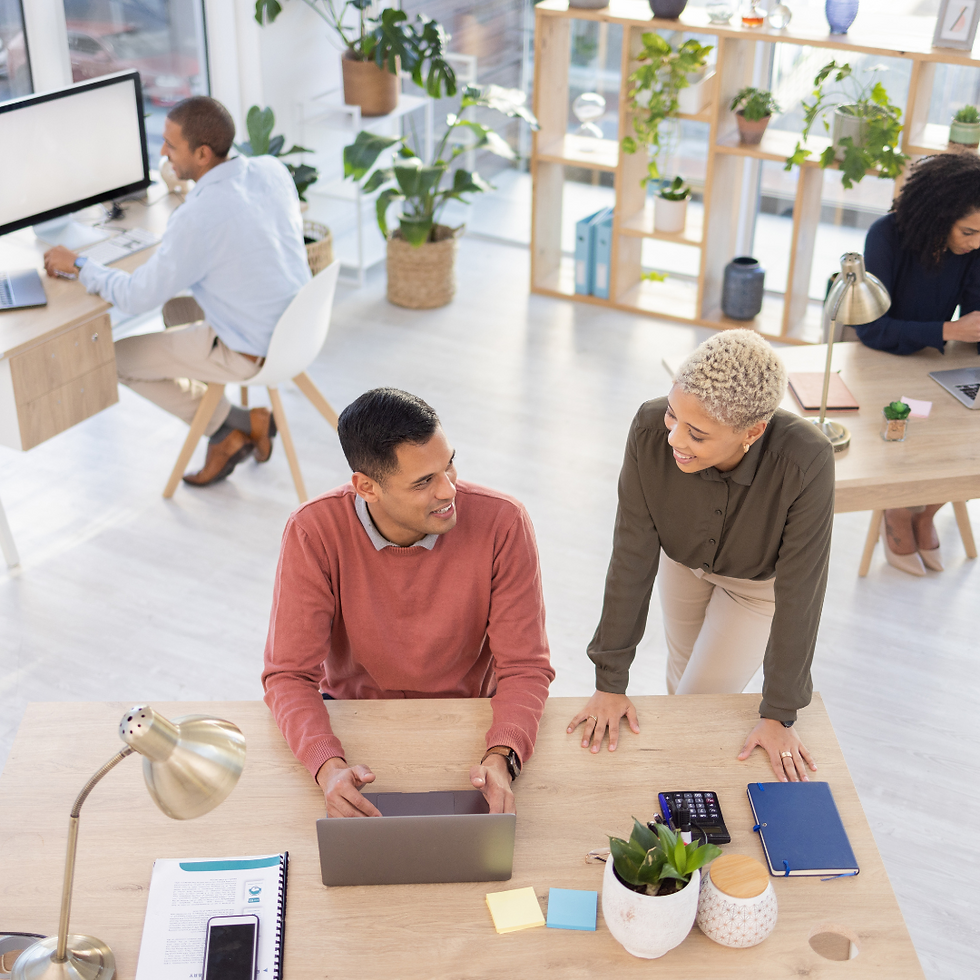
Consumer Rights and Trust
Transparency: Not disclosing the use of AI in design creation could mislead consumers who assume they are purchasing human-designed items.
Personalization vs. Privacy: Striking a balance between offering highly personalized designs and maintaining user privacy is a significant ethical challenge.
Social and Cultural Sensitivity
Cultural Appropriation: AI models might generate designs that inappropriately use elements from various cultures without proper context or credit.
Diversity and Inclusion: Generative AI should be programmed to respect diversity in body types, cultural backgrounds, and various other demographic factors, rather than perpetuating stereotypes or exclusionary practices.
Accessibility
Democratization vs. Monopolization: While AI has the potential to democratize design capabilities, the high costs of entry might also lead to monopolies by a few big players, limiting opportunities for small and independent designers.
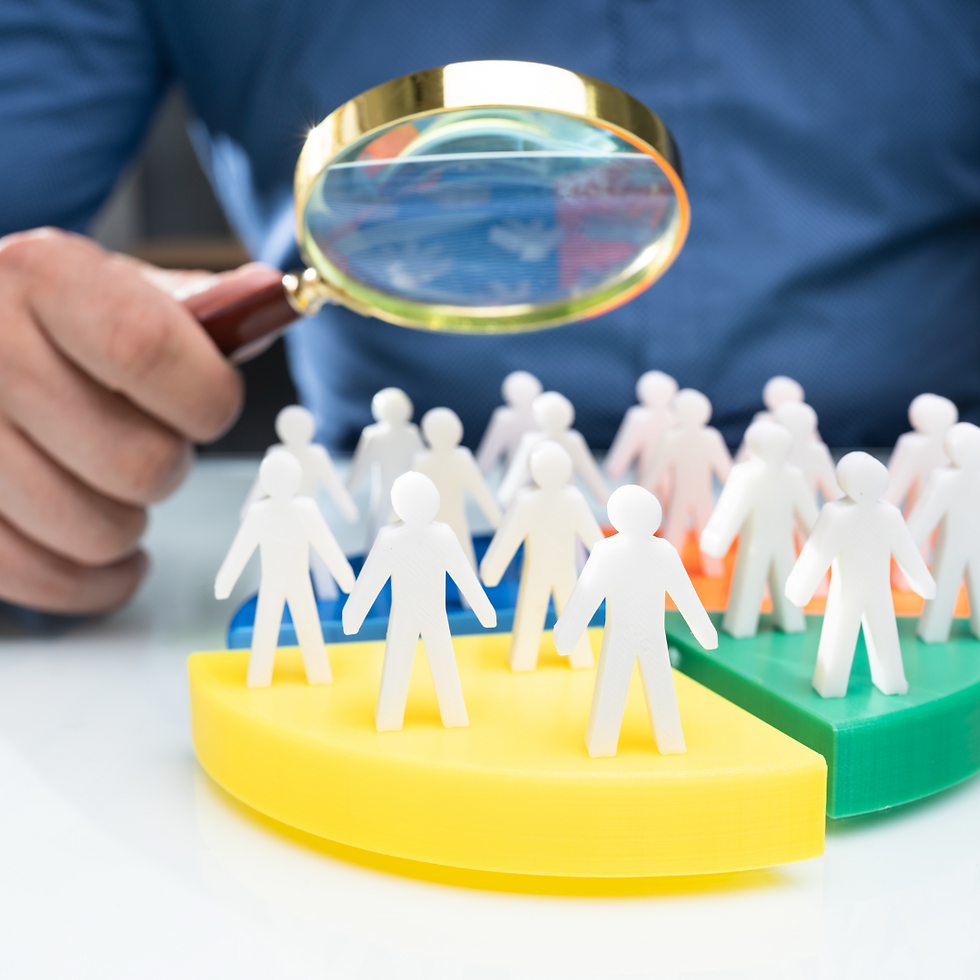
Regulatory Compliance
Legal Frameworks: Existing laws may not adequately cover AI-generated designs, necessitating the creation of new legal frameworks that consider the ethical implications.
Quality & Safety
Quality Control: Over-reliance on AI for quality checks may result in subpar products if the algorithms are not up to the mark. This raises ethical questions about product integrity and consumer safety.
Ethical Business Practices
Fair Competition: The use of advanced AI by major corporations could create an uneven playing field, compromising the principles of fair competition.
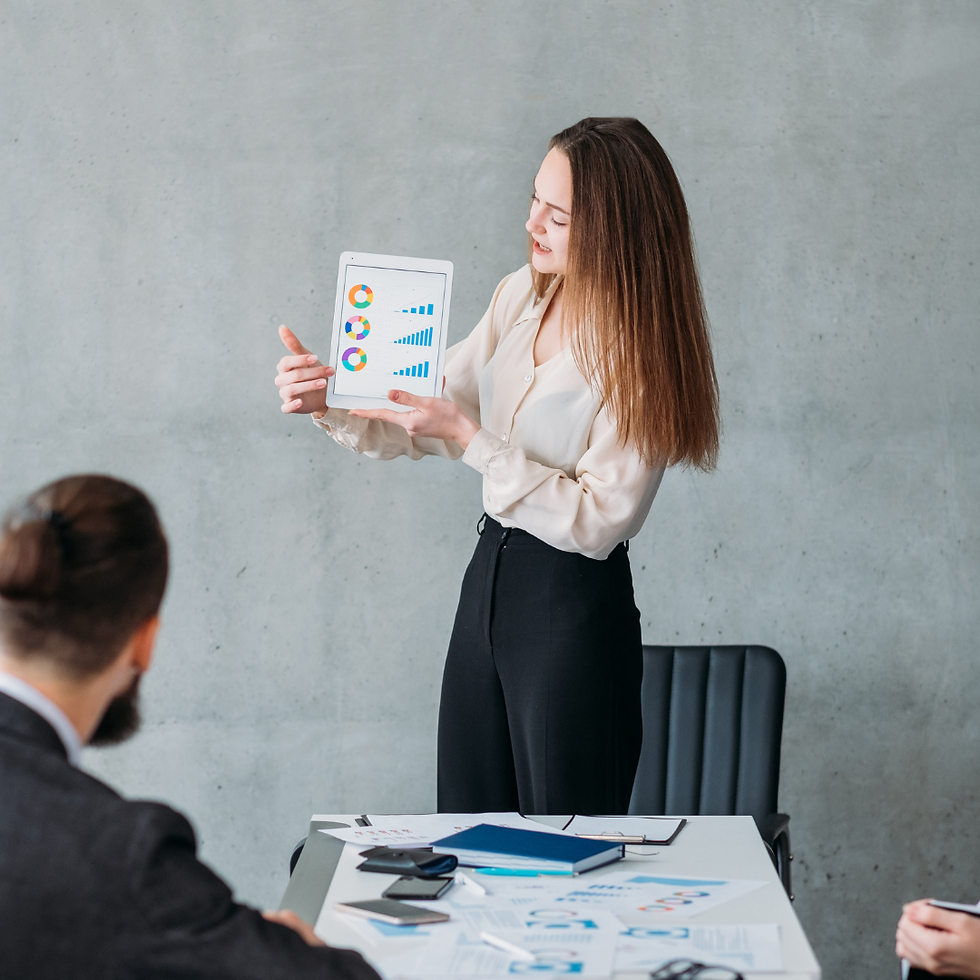
To navigate these challenges, multi-stakeholder dialogues that include fashion designers, AI developers, ethicists, and legal experts may be necessary.
Also, creating industry standards and best practices for ethical AI use in fashion could help mitigate these issues and pave the way for a more ethical and inclusive industry.
The Future of Generative AI
The future of generative AI in the fashion industry appears to be one filled with promise and potential disruptions. Here's a look at some directions this future may take:
Advanced Personalization
Made-to-Order Fashion: AI could offer extreme personalization, from designs tailored to individual consumers' tastes to items optimized for specific body measurements.
Emotion-Driven Fashion: Future AI could even interpret emotional cues from consumers through biometric or behavioral data, customizing designs based on mood or emotional state.
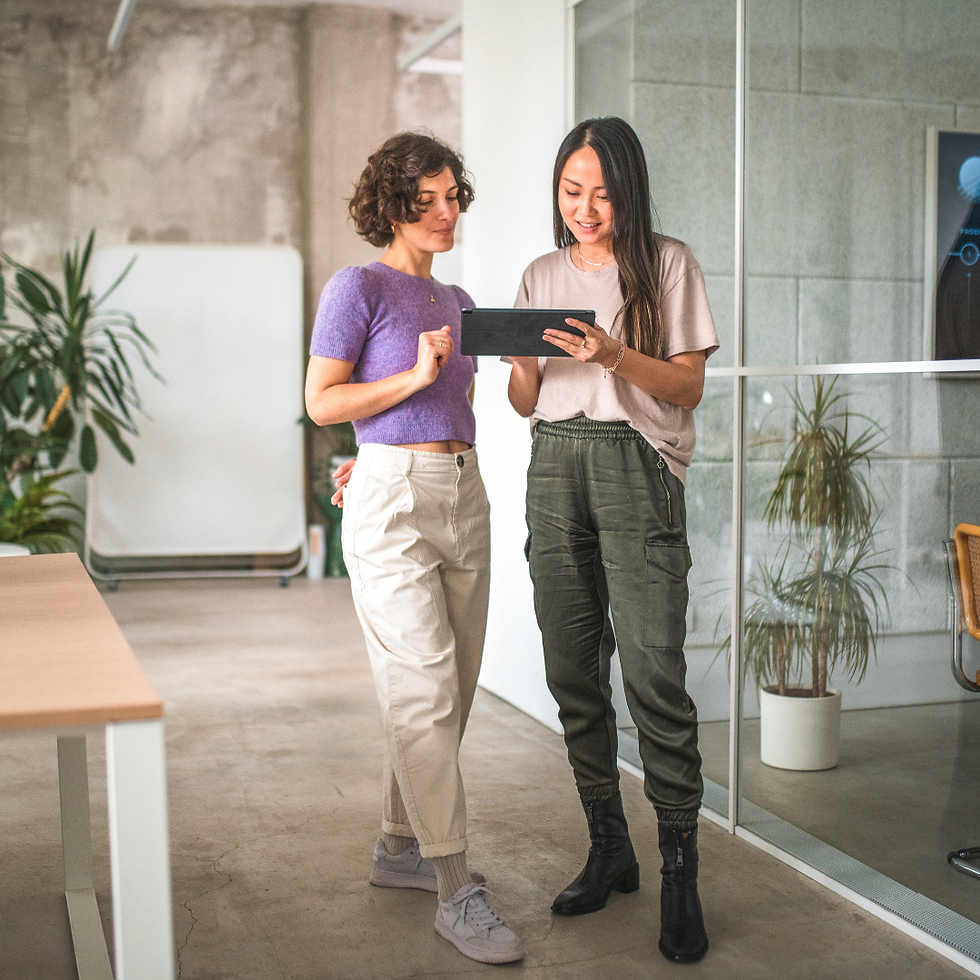
Dynamic Adaptation
Real-Time Trend Analysis: AI algorithms could analyze real-time data to adapt designs dynamically, allowing brands to respond to micro-trends within hours or days.
Seasonless Fashion: With real-time data and quick production cycles, the fashion calendar's seasonal constraints could become obsolete.
Virtual and Augmented Reality Integration
Virtual Fitting Rooms: Generative AI could collaborate with AR and VR technologies to create virtual fitting rooms where customers can try on AI-generated designs.
Virtual Fashion Shows: New collections could be showcased in virtual worlds, possibly even including AI-generated avatars as models.
Sustainable Practices
Closed-Loop Systems: AI could facilitate a circular fashion ecosystem, predicting garment lifecycles, recommending recycling methods, and generating designs that are easier to recycle.
Resource Optimization: From better pattern layouts that reduce material waste to predictive analytics that minimize overproduction, AI can play a pivotal role in making fashion more sustainable.
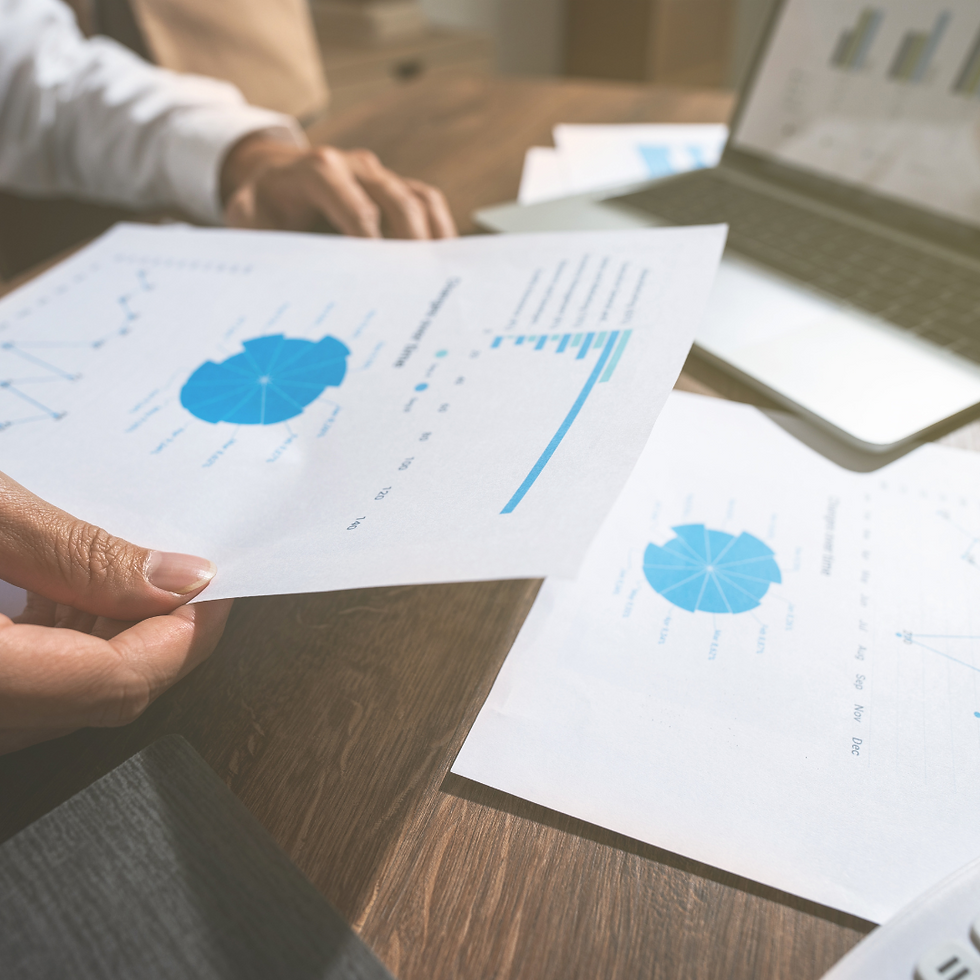
AI Collaborators
AI as a Co-Designer: Designers may work more frequently in tandem with AI, using the technology as a tool for initial drafts and refining AI-generated outputs for final products.
Cross-Industry Collaboration: Fashion brands may collaborate with tech companies, artists, and even customers to create AI-generated designs, fostering a more open and interactive creative process.
Ethical and Regulatory Evolution
Ethical Frameworks: As generative AI becomes more prevalent, ethical guidelines and best practices will likely be developed to navigate issues like copyright, job displacement, and data privacy.
Legislation: As the technology matures, new laws may be enacted to address unique challenges posed by AI, such as intellectual property rights for AI-generated designs.
Niche Markets and Democratization
Bespoke Services for All: Once the preserve of luxury shoppers, bespoke fashion could become widely accessible, thanks to AI's capabilities for customization at scale.
Indie and Small Brands: As AI tools become more accessible and user-friendly, small brands and independent designers will be more empowered to compete with big players.

The Human Element
Retaining Craftsmanship: As generative AI takes on more roles in design and production, a unique selling proposition could become the "human touch," where craftsmanship and emotional resonance are prioritized.
Educational Transformation: Future fashion education may need to incorporate AI literacy as a critical skill for emerging designers, similar to how digital design tools are currently taught.
The confluence of generative AI with other emerging technologies and evolving consumer expectations is likely to radically transform the fashion industry.
However, as with any technological revolution, responsible and ethical implementation will be key to ensuring that the changes benefit both the industry and the consumers it serves.
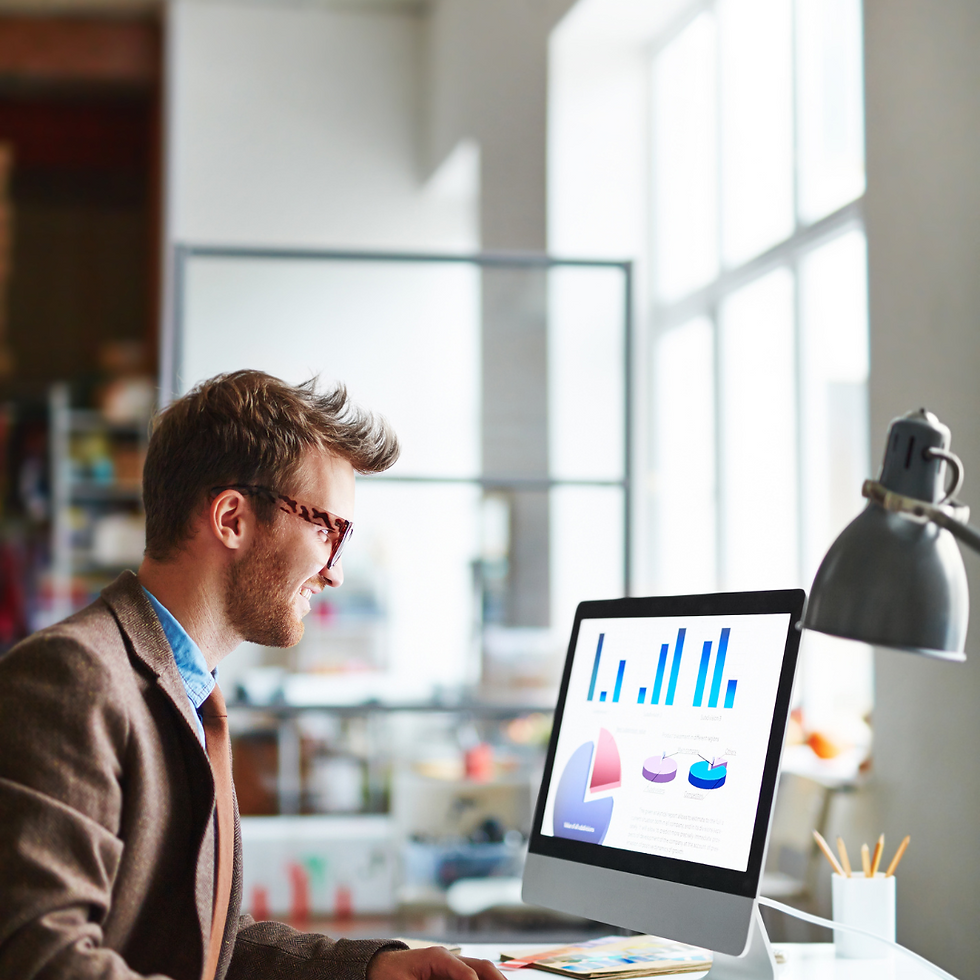
Conclusion:
GenAI can be used to create more personalized and inclusive fashion, more sustainable fashion, and new and innovative business models. As generative AI technology continues to develop, we can expect to see even more innovative and groundbreaking applications of this technology in the fashion industry in the future.
Frequently Asked Questions:
What are some specific ways that fashion brands are using generative AI to forecast trends and analyze market data?
Fashion brands are using generative AI to analyze social media data, customer data, and competitor data to identify trends and opportunities.
Comentarios